Dynamic Pricing for real estate
Dynamic Pricing for real estate
Dynamic Pricing for real estate
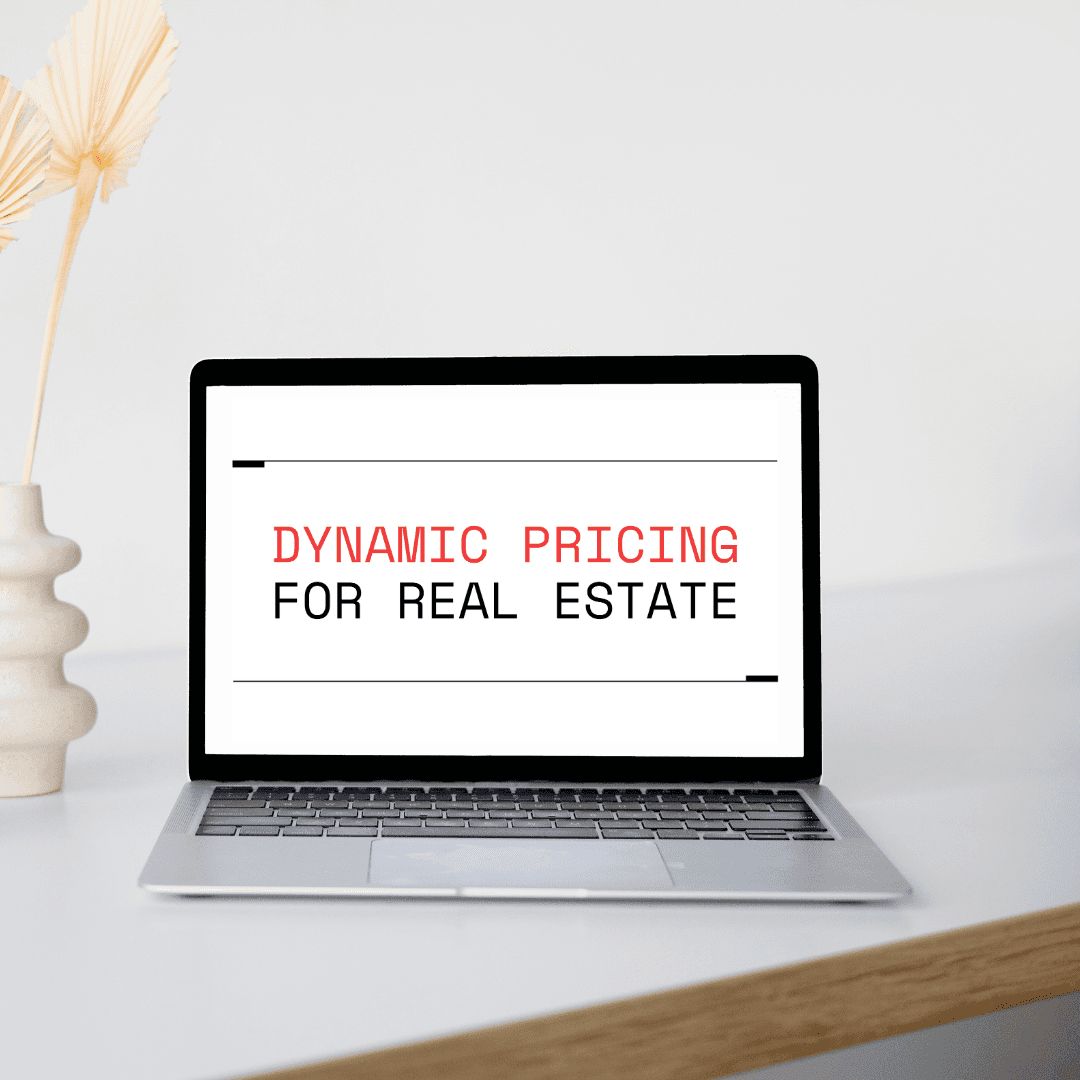
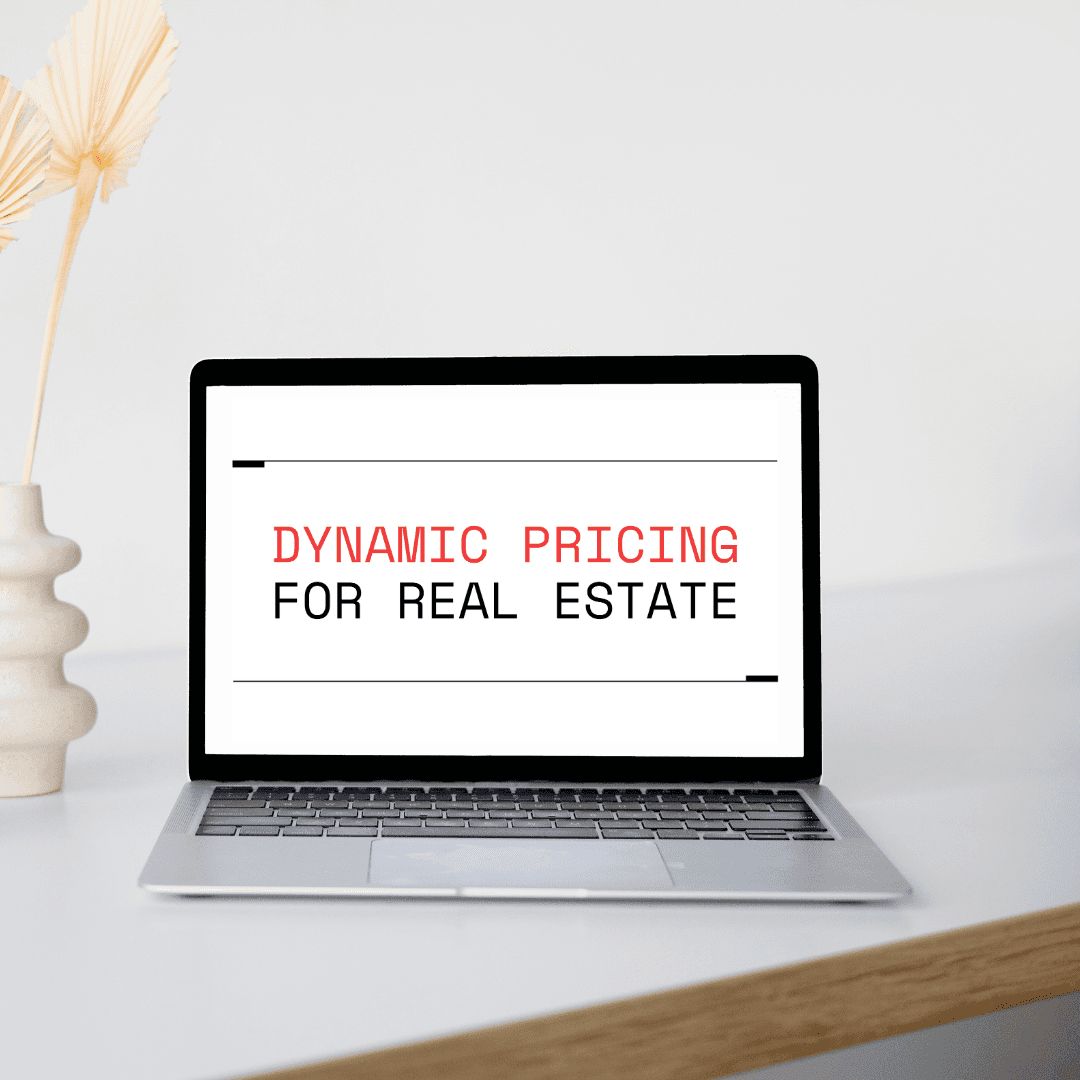
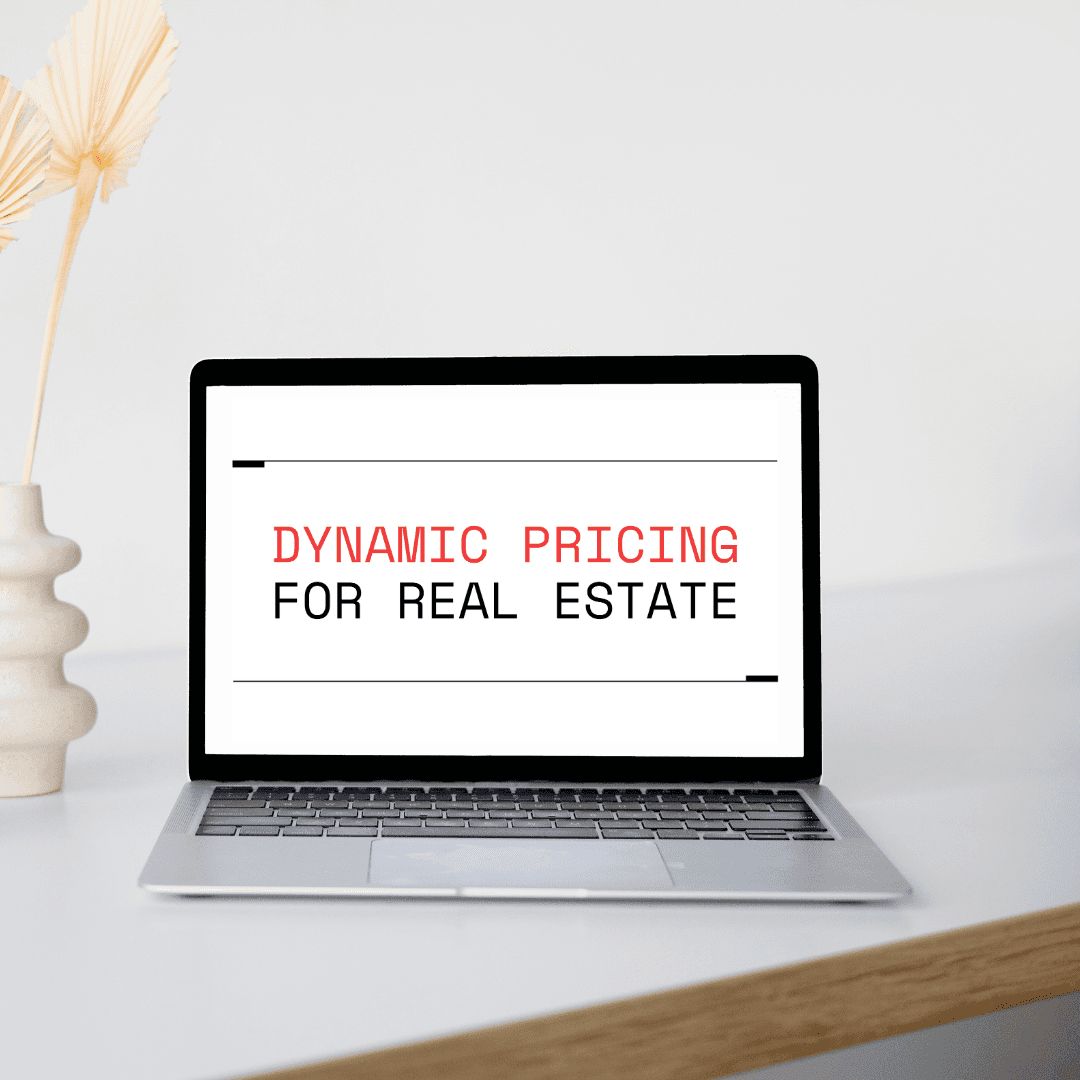
Building Dynamic Pricing for Real Estate
Introduction
Creating a solution for dynamic pricing in the real estate sector was an exciting challenge. The aim was to address inefficiencies and improve transparency in property pricing, leveraging real-time data and predictive analytics.
The Problem
The real estate market has long been plagued by issues such as spurious listings, inflated property prices, and high brokerage costs. In India alone, the total rental pool in residential real estate is estimated at $30 billion, with a $2-4 billion brokerage pool. Static data and lack of market insights often lead to property price discrepancies, causing many properties to remain unsold.
Solution
Dynamic pricing can revolutionize the real estate sector by predicting property values using various data points:
1. Real-time Market Data: Tracking trends and changes in real-time.
2. Historical Data: Identifying patterns over time.
3. Property Features: Considering location, size, amenities, and condition.
4. Comparable Properties: Using data from similar properties.
5. Demand Forecasting: Predicting future demand in specific areas.
Implementation
To develop the dynamic pricing solution, the following steps were taken:
1. Identify Key Data Points: Key factors influencing property values, such as location, size, and features.
2. Develop a Simple MVP: A basic website to capture necessary data and provide initial pricing insights.
3. Test the MVP: Launched in an emerging city to gather data and user feedback.
4. User Acquisition and Feedback: Tracking user engagement and gathering feedback to improve the product.
KPIs for Success
Key performance indicators (KPIs) included:
- Net Promoter Score (NPS): Measuring user satisfaction and likelihood of recommendation.
- Conversion Rate: Tracking the number of users converting to paid services.
- Customer Acquisition Cost (CAC): Calculating the cost of acquiring new users.
Building Dynamic Pricing for Real Estate
Introduction
Creating a solution for dynamic pricing in the real estate sector was an exciting challenge. The aim was to address inefficiencies and improve transparency in property pricing, leveraging real-time data and predictive analytics.
The Problem
The real estate market has long been plagued by issues such as spurious listings, inflated property prices, and high brokerage costs. In India alone, the total rental pool in residential real estate is estimated at $30 billion, with a $2-4 billion brokerage pool. Static data and lack of market insights often lead to property price discrepancies, causing many properties to remain unsold.
Solution
Dynamic pricing can revolutionize the real estate sector by predicting property values using various data points:
1. Real-time Market Data: Tracking trends and changes in real-time.
2. Historical Data: Identifying patterns over time.
3. Property Features: Considering location, size, amenities, and condition.
4. Comparable Properties: Using data from similar properties.
5. Demand Forecasting: Predicting future demand in specific areas.
Implementation
To develop the dynamic pricing solution, the following steps were taken:
1. Identify Key Data Points: Key factors influencing property values, such as location, size, and features.
2. Develop a Simple MVP: A basic website to capture necessary data and provide initial pricing insights.
3. Test the MVP: Launched in an emerging city to gather data and user feedback.
4. User Acquisition and Feedback: Tracking user engagement and gathering feedback to improve the product.
KPIs for Success
Key performance indicators (KPIs) included:
- Net Promoter Score (NPS): Measuring user satisfaction and likelihood of recommendation.
- Conversion Rate: Tracking the number of users converting to paid services.
- Customer Acquisition Cost (CAC): Calculating the cost of acquiring new users.
Building Dynamic Pricing for Real Estate
Introduction
Creating a solution for dynamic pricing in the real estate sector was an exciting challenge. The aim was to address inefficiencies and improve transparency in property pricing, leveraging real-time data and predictive analytics.
The Problem
The real estate market has long been plagued by issues such as spurious listings, inflated property prices, and high brokerage costs. In India alone, the total rental pool in residential real estate is estimated at $30 billion, with a $2-4 billion brokerage pool. Static data and lack of market insights often lead to property price discrepancies, causing many properties to remain unsold.
Solution
Dynamic pricing can revolutionize the real estate sector by predicting property values using various data points:
1. Real-time Market Data: Tracking trends and changes in real-time.
2. Historical Data: Identifying patterns over time.
3. Property Features: Considering location, size, amenities, and condition.
4. Comparable Properties: Using data from similar properties.
5. Demand Forecasting: Predicting future demand in specific areas.
Implementation
To develop the dynamic pricing solution, the following steps were taken:
1. Identify Key Data Points: Key factors influencing property values, such as location, size, and features.
2. Develop a Simple MVP: A basic website to capture necessary data and provide initial pricing insights.
3. Test the MVP: Launched in an emerging city to gather data and user feedback.
4. User Acquisition and Feedback: Tracking user engagement and gathering feedback to improve the product.
KPIs for Success
Key performance indicators (KPIs) included:
- Net Promoter Score (NPS): Measuring user satisfaction and likelihood of recommendation.
- Conversion Rate: Tracking the number of users converting to paid services.
- Customer Acquisition Cost (CAC): Calculating the cost of acquiring new users.